 |
Introduction
Each day, we execute
various acts that we barely acknowledge. Waking up every morning, making and drinking
coffee, getting on the train, and arriving at the office without making any mistakes
seems so simple that performing them requires little conscious thought. Yet the
development of these behaviors is so complex that it is nearly impossible to artificially
engineer.
Daily repetition at the sensory-motor level is, first of all, a key factor in
being capable of diverse and complex behavior. The repeated experience enables
complex behavior by developing gradually a structure following a long consolidation.
Yet, surprisingly, humans sometimes develop creative behavior patterns of which
they have hitherto had no experience in response to certain situations. By what
brain mechanisms are these structured and original creations manifested? Our laboratory
team's goal was to thoroughly examine this question, first by developing an abstracted
neural network model, and then testing the model's hypothesis through experiments
in behavioral psychology and brain physiology.
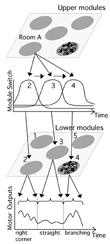
magnified
scene by clicking image
Fig.1 |
Hierarchical
local representation model.
Each of the lower level modules learns independently, and thereby turns a right
corner or moves straight through an intersection, and each of the upper modules
learns sequential combinations of these behaviors. |
|
The
local representation model
In considering
models, it seems reasonable to suppose that complex series of behaviors are composed
of assembly of parts consisting of simpler partial motor primitives. The basic
hypothesis is that first a repertoire of behavioral units that forms the basis
of behavior is acquired. Once these units are assembled, higher level repertoires
develop. The next question is: how can a hierarchically structured mechanism be
constructed on the basis of the neural network model? We thought we could address
this issue by using local neural network modules. In Figure 1, neural network
module groups were divided into two levels. On the lower level, each neural module
continuously learns to be an "expert" in its respective behavioral unit.
For example, in an experiment with robot navigation through several rooms, each
expert module is developed for respective sensory-motor sequence pattern that
corresponds to specific behavior like turning a right corner or passing through
branchies? Then, the lower level modules begin to switch autonomously among them
corresponding with the respective sensory-motor sequence encoded while the robot
explores the room environment. On the upper level, each expert module learns a
specific sequential switching pattern of the lower level modules for specific
behaviors. That is, each upper level module respectively encodes sequences of
events that express a specific route: turning right at a corner, proceeding along
a straight hallway, and then turning a left corner. Basically, a rough scenario
for navigating a specific room is recalled in a specific upper level module network
by which the lower level behavioral module is activated in sequences, then the
actual maneuvering is achieved in accordance with this scenario.
The
distributed representation model
This result was very easy to understand, but the human brain is not limited to
this sort of explicit internal representation.A weak point in this modeling is
that each behavioral unit is mapped to a unique expert module as a one-to-one
mapping manner, which reminds us of an old idea of the "grandmother-cell".By
this, sufficient number of expert modules has to be allocated for covering all
possible different behavioral units.In response to this issue, we have recently
envisaged a hierarchically distributed representation model.
Figure 2 shows the hierarchically distributed representation model. This neural
network model consists of two levels of neural networks, an upper and a lower,
with no module structures in either level. Neural firing activity is slower in
the upper level than in the lower level. There are top-down connections from the
upper level to the lower level network and the upper level neural network switches
the lower level neural activity mode through these connections. For example, when
the upper neural system sends different top-down signal patterns to the lower
network (i.e. two neurons once clamped as 0.8 and 0.2 activities by the top-down
signal, then become clamped as 0.3 and 0.7 activities in Figure 2), the boundary
conditions foractivity in the lower neural network change this creating a switch
in the behavioral motor patterns generated.
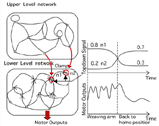
magnified
scene by clicking image
Fig.2 |
Hierarchical
distributed representation model.
The upper neural network is able, by changing the pattern of the top-down signal,
to switch in a diversified manner between behavior patterns that develop in the
lower neural network. |
|
This is a bifurcation,
and is well known in nonlinear dynamics systems. By modulating the top-down signal
patterns, it is possible to generate diverse spatio-temporal patterns from a single
neural network. In fact, after trying out various movement-pattern learning experiments
with an arm-type robot, it became clear that behavioral patterns such as approaching
objects to touch them or cyclical arm waving, and returning the arms to its original
position are self-organized in the lower level network and that each behavioral
pattern can be recalled by modulating the top-down signal patterns.
Another interesting point is that the neural network is also able to develop patterns
other than the movement patterns it has learned. Hybrid-like patterns that interpolate
learned patterns can develop, and sometimes novel and unexpected patterns emerge
from sets of learned patterns. This sort of occurrence is due to the fact that
multiple behavioral patterns are distributed in the memory of a single neural
network. Each of the learned memory patterns is, as it were, embedded in a push-pull
relationship, rather than being maintained independently. When a smooth relationship
is formed among multiple learned patterns in the neuronal phase space, a generalized
structure in which interpolation is possible appears therein. Conversely, if distortion
arises among them, a unique fake memory that has never been experienced may be
represented. The readouts from these fake memories provide opportunities for the
creation of diverse behaviors. Learning is, therefore, the development of a kind
of global structure (gestalt), and the fact that generalizations and diversification
coexists is of deep interest.
Conclusion
In this article, I have introduced two different scenarios for the structural
development of behavior. The former scenario, if anything, takes the reductionist's
perspective that the whole is resolved into explicit portions and is easy to describe
objectively. However, one senses that it does not sufficiently account for complex
human phenomena. In the latter scenario, the relationships alone exist. It is
assumed that a global structure emerges as the results of interactions among the
parts. This holistic position can sufficiently represent interesting aspects of
the human mind. However, this theory is far from complete. In the future, we would
like to move between the reductive and holistic positions, focusing on modeling
research, to determine whether a more complete scenario can be developed for the
learning and development of behavior in the brain. |
 |
 |
|
 |
|
|