  |
 |
 |
Interaction of neural activities and
information geometry
Laboratory for Mathematical Neuroscience
|
|
 |
The functions of our brains are accomplished by the activities of numerous nerve cells. Therefore, to understand the functions of our brain, we must understand the interaction of neural activities. Yet, understanding this interaction is easier said than done. A new approach is required to properly grasp the nature of the interaction. We proposed a mathematical framework, and hence a data analysis method, to study the interaction of neural activities (1).
But why is a new approach needed? The answer is simple. Human intuition is often inaccurate and, when many variables (i.e. neurons) are involved, unable to fully understand the interactions. By recognizing and reorganizing phenomena mathematically, we can refine our intuition and develop a more precise understanding, leading to new analytical methods. Once new methods are developed, they may be employed by others.
The mathematical framework of information geometry is used to develop this method. Information geometry helps us refine our intuition and resolve, through its intuitive format, a wide range of problems in various disciplines including statistics, information theories, control systems and learning mechanisms. To better understand this "intuition", consider two simple examples below.
Figure 1 (left) shows datum points in three dimensions. Each point indicates the values of three variables (e.g. the blue point stands for (X, Y, Z)=(1,1,0)). If you only look at these points as a pair of variables, that is, only in a two-dimensional way, the three-dimensional structure is lost (Fig. 1, left). In examining the interaction of neural activities, we often investigate only the interaction between two neurons. However, as the simple example in Fig 1 indicates, the numerous interactions between nerve cells may involve structures having dozens, or even hundreds, of dimensions. This fact makes it necessary to move beyond investigating only pair-wise interactions.
Now, take a look at Figure 2. Under two different experiment conditions, different interactions within a group of neurons are observed. Those interactions are the two datum points, P and Q (please note that the difference is represented in two-dimension in the figure, even though the actual dimension in data can be several dozen dimensions). The distances between these datum points are measured separately for their impacts on the X and Y axis. Conventional analysis of nerve cell interactions produces obliquely angled coordinates of X and Y as shown in the right part of Figure 2. By contrast, our analytical method generates right angles to the X and Y axis as shown in the left part of Figure 2. Thus, our method, not the conventional analysis, can utilize the Pythagorean theorem to precisely decompose each impact on X and Y (i.e. A and B, respectively in Fig. 2, left). More precisely, this geometrical structure lies in probability space where the X and Y axis are not straight lines but curves. This is an essential property of the probability space, and cannot be corrected. Our methods construct the orthogonal system in such a space.
In recent years, measuring techniques that are used in studies of the brain have experienced rapid improvements. For example, until recently a technique to record nerve cell activity that used electrodes had a maximum range of several tens of cells, but the potential range is expanding and will soon be able to record the activity of hundreds of cells. Our method can crack the data generated from such methods, and provide interpretations of precise details in a consistent manner. Furthermore, experiment data retrieved from a variety of advanced techniques will eventually require the analysis of numerous interactions. As our method is general, it is applicable to many of these situations, although with some limitations. Our method has been successfully applied to DNA microarray data and revealed the higher-order interaction in gene networks (2).
We are currently collaborating with several laboratories to analyze experiment data using this method. Given that brain functions happen through the interactions of neural activities, they are likely to form flexible, functional assemblies for specific purposes and times. Object recognition, for example, requires several cells groups to coordinate input to be able to trigger a response. There are many different cell groups reacting to various colors, shapes, and motions. These reactions must be coordinated. We hope these orchestrated interactions can be investigated more thoroughly by employing our analytic method. Finally, we appreciate the outstanding environment provided by RIKEN BSI, including the daily discussions with many excellent experimental researchers and the use of multi-electrodes data from Dr. Earl Miller's laboratory at the RIKEN-MIT Center.
(1)Nakahara, H., & Amari, S. (2002)Information geometric measure for neural spikes. Neural Computation. 14(10); pp. 2269-2316
(2)Nakahara et al.(2003)Gene interaction in DNA microarray data is decomposed by information geometric measure. Bioinformatics. 19(9); pp. 1124-1131. |
|
 |
Figure 1 |
 |
|
 |
Figure 2 |
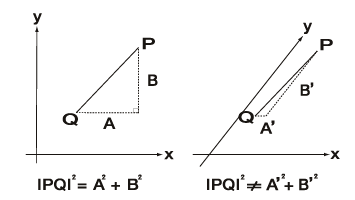 |
|
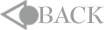 |
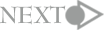 |
|
|