Introduction
The Open Information Systems Laboratory has general interest in intelligent blind and sparse signal processing and nonlinear system theory approaches in computational neuroscience, with the emphasis on artificial neural network models with underlying components (processing units) and self-organizing behavior. Underlying this interest is a belief that biologically plausible artificial neural systems and associated learning algorithms play key roles in understanding information processing problems involved in advanced brain functions and neural networks mechanisms.
One of our objectives is the development of novel and advanced blind and sparse signal processing algorithms and tools, such as robust Principal Components Analysis (PCA), Minor Components Analysis (MCA), Independent Components Analysis (ICA), Multichannel Blind Deconvolution (MBD), Higher Order Statistics (HOS), Nonlinear Adaptive Filtering (NAF) and Joint Time-Frequency (JTF) methods to analyze multi-sensory (multi-electrode) biomedical signals, especially electroencephalographic (EEG), magnetoencephalographic (MEG) and electromyographic (EMG) data.
We need novel and innovative techniques that will be referred to as intelligent blind signal processing (IBSP) since brain sources are "blind" in the sense that they are unidentified, extremely weak, not stationary, distorted by noise and other interferences. Furthermore, these sources are distributed and change their locations over time.
The generalized IBSP problem can be formulated as follows (see Fig.1). We have available only records of measured noisy signals from an unknown MIMO (multiple-input multiple-output) nonlinear dynamic mixing system referred as the mixing system or environmental model. The objective is to find an inverse system, termed a neural network model or an adaptive inverse system, in order to estimate the primary input source signals and their number. This estimation can be performed only on the basis of the sensor (mixed) signals as well as some very limited a priori knowledge about the mixing and filtering system. Preferably, it is required that the inverse system should be self-adaptive and self-organized, so that it has some tracking capability in non-stationary environments [1, 2]. In other words, it is required that the inverse system should have some intelligent features, in the sense that it has self-organized abilities in order to estimate not only the number of original source signals but also their waveforms, stochastic properties and localize them dynamically in the space in non-stationary and noisy and unpredictable environment.
The problems of separating and estimating the original sources from the sensor array without knowing the transmission channels characteristics can be formulated as a number of simpler and closely related sub-problems: Independent Components Analysis (ICA), Blind Source Separation (BSS), Blind Signal Extraction (BSE), Multichannel Blind Deconvolution (MBD) and Blind Equalization (BE).
There appears to be something magical about blind signal processing; we are estimating the original source signals without knowing the parameters of mixing and/or filtering processes. It is difficult to imagine that one can estimate this at all. In fact, without some a priori knowledge it is not possible to uniquely estimate the original source signals. However, IBSP preserves waveforms of original sources.
For such applications, it is essential to develop reliable, robust and effective learning algorithms which enable us to extract and localize only several specific brain source signals that are potentially interesting and contain useful information. One of our current objective is to develop novel approaches and adaptive learning algorithms that are more robust with respect to environmental noise, than available algorithms and models. Several new approaches have been already successfully applied to the elimination of noise under the assumption that the reference noise is available [1, 2]. Another problem which we currently investigating is, the development of more sophisticated nonlinear models of mixtures and un-supervised filtering. Theoretical aspects of these research projects are investigated in collaboration with Professor Amari and His Laboratory for Information Synthesis [1, 3, 4].
On basis of IBSP and other advanced unsupervised learning algorithms we intend in the near future to develop and design intelligent electronic devices or machines that similar to human being will be able to have selective attention, enhance speech and recognize voices, odors or human faces.
Potential Applications BSP in EEG/MEG Data Processing
The problems of blind signal processing have many potential applications in various fields such as biomedical signal analysis and processing, geophysical data processing, data mining, wireless communications, speech and image recognition and enhancement, etc.
To understand human neurophysiology, we rely on several types of non-invasive neuroimaging techniques including EEG, MEG and fMRI.
|
- |
magnified scene by clicking image
Fig. 1 | Conceptual diagram illustrating blind signal processing or blind identification problem |
|
Neural activity in the cerebral cortex generates small electric currents which create electric potential fluctuations on the surface of the scalp (detected by EEG) as well as very small magnetic fields which can be detected using SQUIDs (SuperConducting QUantum Interference Devices). The greatest benefit of MEG is that it provides information that is complementary to EEG. In addition, the magnetic fields (unlike the electric potentials) are not distorted by the intervening biological mass and low-pass filtering effects. Under certain circumstances, this allows for the precise localization of the neural currents responsible for the magnetic field.
If one knows the positions and orientations of the sources in the brain, one can calculate the patterns of electric potentials or magnetic fields on the surface of the head. This is called the forward problem. If instead all one has only the patterns of electric potential or magnetic fields then one must try to calculate the locations, orientations and wave forms of the sources. This is called the inverse problem. Inverse problems are notoriously more difficult to solve than forward problems. In this case, given only the electric potentials and magnetic fields on the surface there is no unique solution to the problem. The only hope is that there is additional information available that can be used to constrain the infinite set of possible solutions to a single unique solution. This is where Intelligent Blind Signal Processing will be useful. The idea is that one must use all the information available to solve the problem. It appears that techniques and methods of blind source separation (BSS) are related to those currently used in electromagnetic source localization (ESL). This framework provides a powerful methodology by which several different types of information can be combined to aid in making inferences about a problem.
In Fig. 2, we show three neural sources, represented in this case by equivalent current dipoles, in the cortical grey matter of the brain. The electrodes or sensors on the head's surface detect the electric potential differences due to the extracellular currents generated by these active sources. The arrows merely demonstrate that each electrode detects some of the current flow from each neural source. The currents do not flow directly from the sources to the electrodes, but instead flow throughout the volume of the entire head.
|
- |
magnified scene by clicking image
Fig. 2 | Noninvasive multi-electrodes recording of activation of the brain using EEG and/or MEG; Schematic illustration of dipolar model of brain sources with overlapping projection to the scalp |
|
Recorded sensor signals are weak, non-stationary and distorted by noise and other interferences. Our primary objective is to develop and use IBSP algorithms as preprocessing tools in order to reduce noise and other interferences like artifacts [2, 3, 4]. Our second objective is to use the IBSP as a tool to localize the brain sources and investigate the flow of information in the brain for specific mental tasks or situations.
Fig. 3 illustrates exemplary promising application of blind source separation and independent component analysis (ICA) algorithms for extraction and localization of brain source signals. In the MEG experiments performed in collaboration with Helsinki of University of Technology, Finland, the stimulus presented to the subject, was produced by a sub-woofer, and conveyed to the shielded-room via a plastic tube, ending in a balloon [2].
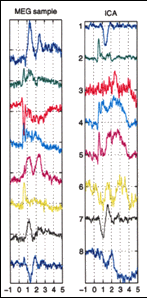 |
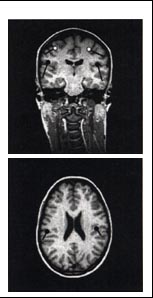 |
- |
Fig. 3 |
(a) |
A subset of the 122-MEG channels |
(b) |
The first strongest 8 independent components (IC-extracted sources) of the MEG data |
(c) |
The superposition of the localizations of the dipole originating IC1 (black circles, corresponding to the auditory cortex activation - AEF) and IC2 (white circles, corresponding to the somatosensory SI cortex activation - SEF) onto magnetic resonance images (MRI) of the subject [2] |
|
[References]
- S. Amari and A. Cichocki: Adaptive blind signal processing - neural network approaches, Proceedings IEEE (invited paper), Vol. 86, No. 10, pp.2026-2048, Oct. (1998)
- A.Cichocki, J. Karhunen, W. Kasprzak and R. Vigario: Neural networks for blind separation with unknown number of sources, Neurocomputing, Vol. 24, pp.55-93 (1999)
- O. Jahn, A. Cichocki, A. Ioannides and S. Amari: Identification and elimination of artifacts from MEG Signals using efficient Independent Components Analysis, BIOMAG-98, Sendai, JAPAN, Aug. (1998)
- A. Cichocki, J. Cao, S. Amari, N. Murata, T. Takeda and H. Endo: Enhancement and blind identification of magneto-encephalographic signals using independent component analysis, BIOMAG-98, Sendai, JAPAN, Aug. (1998)
|